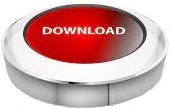
arXiv preprint arXiv:2010.11929 (2020)Įsser, P., Rombach, R., Ommer, B.: Taming transformers for high-resolution image synthesis. arXiv preprint arXiv:1810.04805 (2018)ĭosovitskiy, A., et al.: An image is worth 16x16 words: transformers for image recognition at scale. PMLR (2020)ĭevlin, J., Chang, M.W., Lee, K., Toutanova, K.: BERT: pre-training of deep bidirectional transformers for language understanding. In: International Conference on Machine Learning, pp. Ĭhen, M., et al.: Generative pretraining from pixels. In: Vedaldi, A., Bischof, H., Brox, T., Frahm, J.-M. 7291–7299 (2017)Ĭarion, N., Massa, F., Synnaeve, G., Usunier, N., Kirillov, A., Zagoruyko, S.: End-to-end object detection with transformers. In: Proceedings of the IEEE Conference on Computer Vision and Pattern Recognition, pp. In: NeuPIS (2021)Ĭao, Z., Simon, T., Wei, S.E., Sheikh, Y.: Realtime multi-person 2D pose estimation using part affinity fields. (TOG) 33(4), 1–10 (2014)Ĭao, C., et al.: The image local autoregressive transformer. Ĭao, C., Hou, Q., Zhou, K.: Displaced dynamic expression regression for real-time facial tracking and animation. In: Ferrari, V., Hebert, M., Sminchisescu, C., Weiss, Y. 187–194 (1999)Ĭai, H., Bai, C., Tai, Y.-W., Tang, C.-K.: Deep video generation, prediction and completion of human action sequences. In: Proceedings of the 26th Annual Conference on Computer Graphics and Interactive Techniques, pp. arXiv preprint arXiv:1703.10717 (2017)īlanz, V., Vetter, T.: A morphable model for the synthesis of 3D faces.

īerthelot, D., Schumm, T., Metz, L.: Began: boundary equilibrium generative adversarial networks.
Extensive experiments on human motion datasets validate the efficacy of our model.īansal, A., Ma, S., Ramanan, D., Sheikh, Y.: Recycle-GAN: unsupervised video retargeting. The guided information is represented as a pose coordinate sequence extracted from the driving videos. Particularly, our QS-Craft employs transformer in its structure to utilize the attention architectures. The key novelties come from the newly introduced three key steps: quantize, scrabble and craft. To this end, this paper proposes a novel model of learning to Quantize, Scrabble, and Craft (QS-Craft) for conditional human motion animation. Despite the success of Generative Adversarial Network (GANs) methods in image and video synthesis, it is still very challenging to conduct cHMA due to the difficulty in efficiently utilizing the conditional guided information such as images or poses, and generating images of good visual quality.
Given a source image and a driving video, the model should animate the new frame sequence, in which the person in the source image should perform a similar motion as the pose sequence from the driving video. This paper studies the task of conditional Human Motion Animation (cHMA).
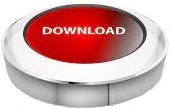